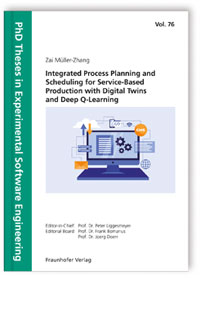
PhD Theses in Experimental Software Engineering, Band 76
Zai Müller-Zhang
Hrsg.: Peter Liggesmeyer, Frank Bomarius, Jörg Dörr; Fraunhofer IESE, Kaiserslautern
2025, 158 S., num. illus. and. tab, Softcover
Sprache: Englisch
Kaiserslautern, RPTU Kaiserslautern-Landau, Diss., 2024
Fraunhofer Verlag
ISBN 978-3-8396-2054-0
Inhalt
Scheduling complex production processes in real time is a challenging task because it typically takes hours to find optimal schedules. In recent years, reinforcement learning (RL) has shown great potential for solving complex scheduling problems. An appropriately trained RL agent can quickly respond to similar situations with near-optimal strategies to achieve good enough or even brilliant performance.
This work presents an efficient methodology to apply the deep Q-learning algorithm to integrated process planning and scheduling. The presented RL methods were proven to be efficient in finding near-optimal schedules in real time. Meanwhile, the trained RL agents show great flexibility in handling process deviations without sacrificing production performance.
Verfügbare Formate
Computing, Information Technology, Applied computing, Computer applications, Artificial intelligence, Operation Research,
* Alle Preise verstehen sich inkl. der gesetzlichen MwSt. Lieferung deutschlandweit und nach Österreich versandkostenfrei. Informationen über die Versandkosten ins Ausland finden Sie hier.