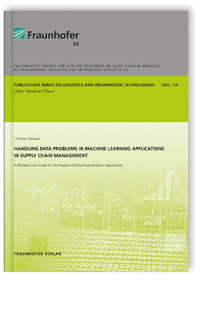
A Multiple-Case Study on the Analysis of Data Augmentation Approaches
Schriftenreihe Logistik und Informationstechnologien / Publication series on logistics and information technologies, Band 10
Christian Menden
Hrsg.: Alexander Pflaum; Fraunhofer-Arbeitsgruppe für Supply Chain Services des Fraunhofer IIS
2021, 365 S., num., mostly col. illus. and tab., Softcover
Sprache: Englisch
Bamberg, Univ., Diss., 2021
Fraunhofer Verlag
ISBN 978-3-8396-1786-1
Inhalt
In recent years, considerable progress has been made in research on artificial intelligence, particularly in the sub-area of machine learning (ML) where information is extracted from huge data sets. In practice, however, the existing data is often dirty, erroneous, not available in sufficient quantity, or does not meet the requirements for a direct application of ML methods. Against this background, data augmentation (DA) methods can be used to improve the data quality with the aim of enabling an initial application of ML methods or improving the results of existing ML models. Today, there is a wide range of different DA methods, which makes it oftentimes difficult to select an appropriate DA method for a particular application. Further, it remains unclear what the potential benefits and possible obstacles are to using DA for ML methods in practice. In this regard, this dissertation aims to contribute to a better understanding of DA and to demonstrate, by means of a multiple-case study, how DA can improve the performance and applicability of ML methods in the context of supply chain management.
Verfügbare Formate
Fraunhofer SCS, Fraunhofer IIS, Machine Learning, Applied Mathematics, Transport Studies, Data Augmentation, Business mathematics and systems,
* Alle Preise verstehen sich inkl. der gesetzlichen MwSt. Lieferung deutschlandweit und nach Österreich versandkostenfrei. Informationen über die Versandkosten ins Ausland finden Sie hier.